2023 Budget & Planning in Uncertain Times
Share this article:
Rose Report: Issue 59
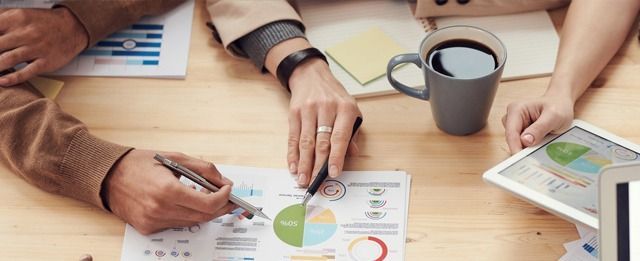
By Ted Rose, President and CEO, Rose Financial Solutions
It’s that time of the year when company leaders develop their annual budgets to project income, profits, and returns on investments and control expenses for the coming year. While this yearly exercise of creating a roadmap to manage financial expectations and goals for the coming year is challenging, it will be even trickier this year due to the current economic downturn, unpredictable workforce trends, and supply chain challenges.
How is the unpredictable impact of the pandemic affecting corporate budgeting decisions? To explore current budgeting trends across industries, Forrester’s Budget Pulse Survey, 2022, polled 382 U.S. business and technology decision-makers to assess current expenditure projections for 2023. According to the survey:
- Most decision-makers expect at least some increase in overall spending in the next 12 months; less than 10% expect a decrease.
- On average, 60% of those surveyed expect to increase spending on personnel, and 62% expect to increase spending on external services.
- Of those surveyed, 67% said their technology investments would continue to grow.
As these survey results reveal, many business decision-makers have a positive outlook for 2023. While optimism is essential to growing a company, you must establish realistic growth expectations that align with your company’s goals and financial standing. When preparing your 2023 budget, it’s essential to keep the following in mind.
- Make sure you create a cost structure that matches your growth expectations based on the market, the economic outlook, and your previous year’s budget.
- Confirm that staffing levels correspond to revenue levels. To maintain an accurate forecast, you must monitor income growth and other expenses, such as staffing. It is expensive and risky to make hiring decisions or other investments based on unrealistic projections.
- Often, billing occurs at the end of the month; however, you’ll have costs you’ll need to pay throughout the month. In anticipation of your cash needs, make sure to project the amount of cash necessary to maintain operations before you receive revenue. Plan for at least 45-60 days of money to cover your expenses. This is best accomplished by projecting your balance sheets and cash flow statements for the upcoming year.
- Make sure to include your senior leadership and managers in your budget planning. Operating managers should calculate their divisions’ projected sales revenues, operating costs, and gross profits. Giving them the responsibility to build out their plans motivates them and helps you hold them accountable for meeting those numbers or raising their hands early when unexpected results occur. While it’s wise to encourage them to take reasonable risks from the bottom up, the view from the top down should be more conservative. Remember that setting overly ambitious goals for your entire company can throw off budget planning for each department.
- Monitor your annual budget regularly—either monthly or quarterly, to see if your forecasts are on track with actual performance. Prepare and share monthly reports illustrating the projections and actual results. By analyzing your numbers, your team can adjust as needed to keep their goals on track.
As we head into 2023, it is more important than ever to have financial clarity to make sound business decisions. During uncertain times, it’s critical to have a financial planning system that enables decision-makers to recognize when budgeted projections are veering off course so they can act to pivot rapidly and get back on track.
Schedule an introductory virtual meeting to find out how RFS can provide you with the financial clarity needed to succeed.
This content is for information purposes only and should not be considered legal, accounting or tax advice, or a substitute for obtaining such advice specific to your business.
The post 2023 Budget & Planning in Uncertain Times appeared first on Rose Financial Solutions.
Visit Us On:
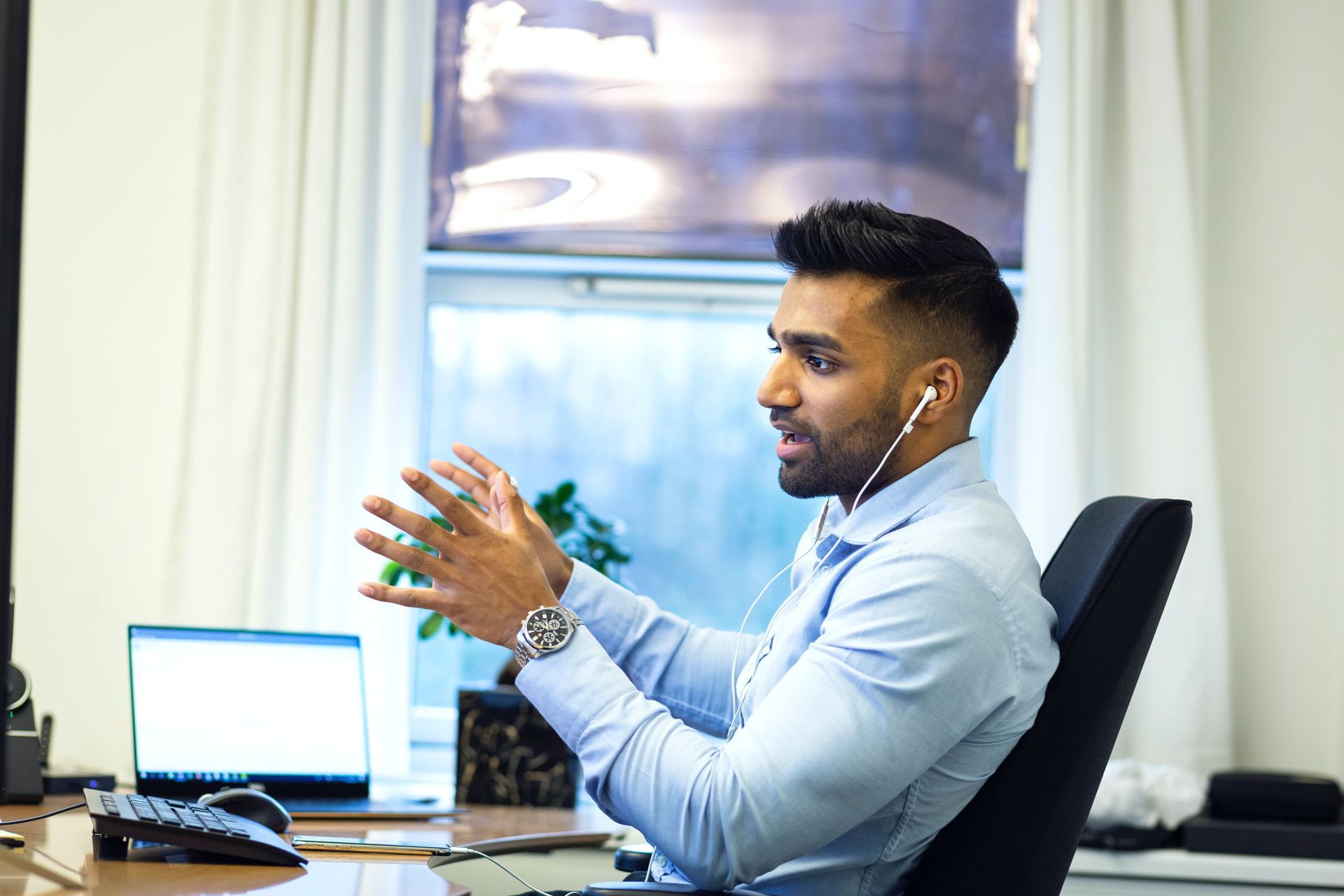
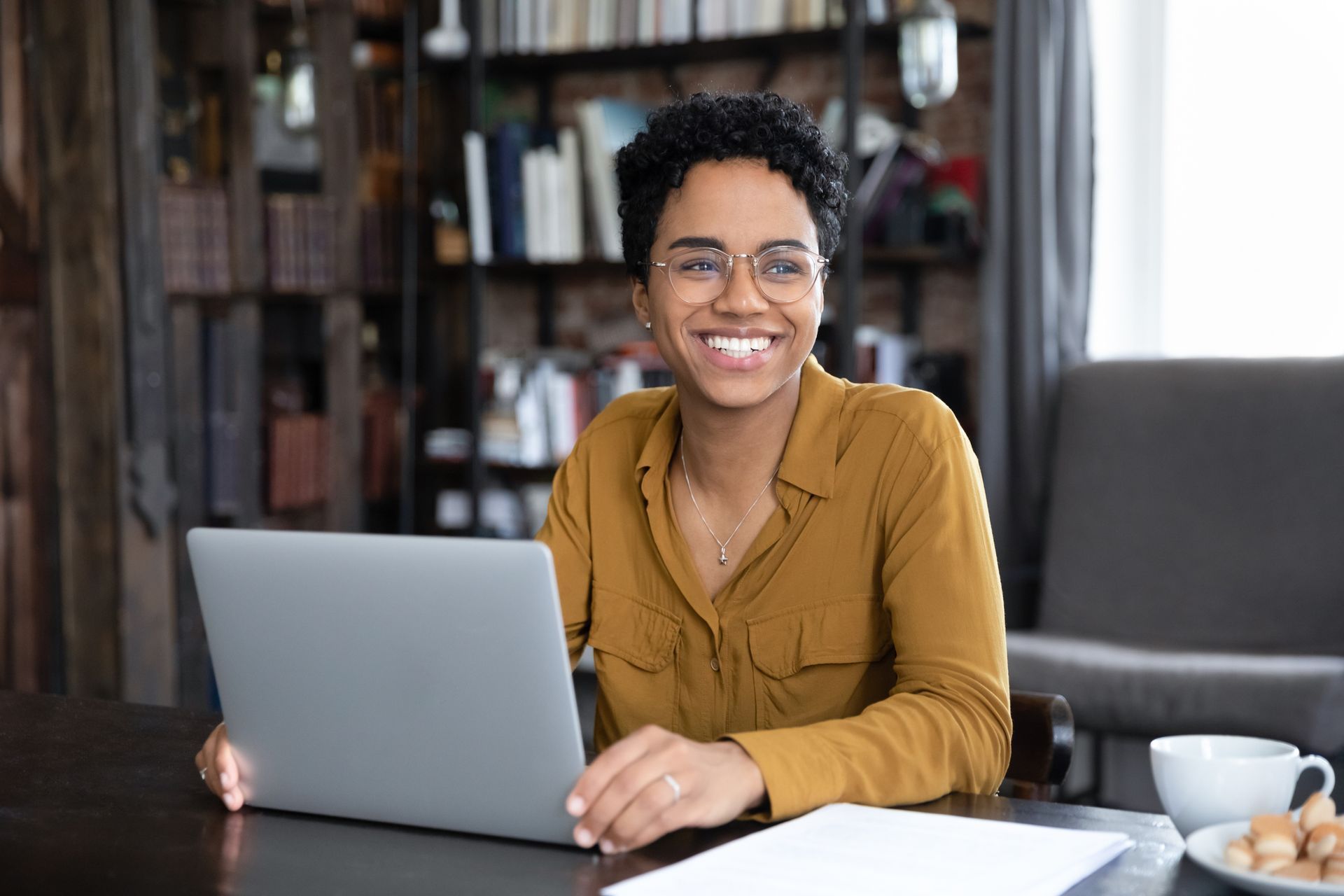